Calculations of statistical power and the related values of sample size and smallest detectable effect size are essential to the design of high-quality population health studies. However, conducting these calculations can be tricky. In evaluating proposals, we find that power calculations are among the most common difficulties in E4A applications. The goal of this Methods Note is to review the key inputs for conducting power calculations to ensure proposed studies in population health research are adequately powered and evaluated fairly.
by Ellicott C. Matthay, PhD
Published January 15, 2020
If the sample size is already determined, for example if the data have already been collected or only a specified number of people could possibly be included in the study, researchers should present estimates of the smallest detectable effect size (i.e., the smallest effect size which the study design is likely to be able to detect). If the sample size is to be determined, researchers should provide a justification for the proposed sample size based on the expected magnitude of the effect of the treatment.
Typically, the following information should be used and reported in the calculation:
- The unit of analysis (individuals, neighborhoods, classrooms, etc.)
- The sample size (if set in advance) or the expected magnitude of the effect of the exposure or treatment (if the sample size is not imposed in advance)
- If data are clustered:
- By place (e.g., measures on individuals in the same school or neighborhood), the number of clusters as well as the number of people in a cluster
- By time (e.g., repeated measures on the same individuals), the number of people as well as the number of repeated observations on the same person
- The magnitude of correlation between observations in the same cluster (i.e., the intra-class correlation)
- How the analysis will account for the clustered observations
- The distribution of exposure (or treatment):
- If exposure is binary, the fraction of people exposed
- If exposure is continuous and approximately normally distributed, the standard deviation
- The assumed type 1 error rate (typically no higher than 5%)
- Whether a one-tailed or two-tailed statistical test was used, and if a one-tailed test is used, justification for this choice
- If using instrumental variables, regression discontinuity, differences-in-differences, or a similar analytic approach:
- The strength of the association between the instrument (or the regression discontinuity) and treatment variable, expressed as an F-test or similar and as an r2 calculation or similar (e.g., the fraction of the variance in the treatment that is determined by the instrument)
- The statistical power the analysis aims to achieve (we recommend calculations based on 80% and 90% power)
- The distribution of the outcome (e.g., the incidence rate, or the mean and standard deviation) to help contextualize the smallest detectable effect size in the proposed study
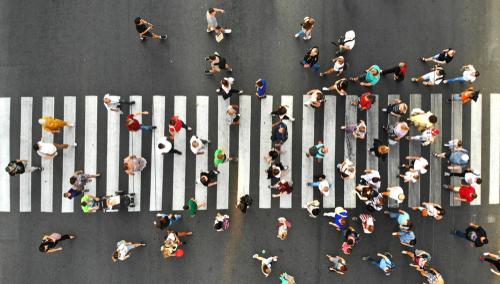
Calculations should report on the assumed values of these parameters and any data or prior research on which they are based. Because there is usually some uncertainty about the assumptions used to guide these calculations, it is more convincing to show the calculations for a range of input assumptions. If the study entails subgroups or tests of differential effects, provide a power calculation corresponding with at least (1) the most important analysis and (2) for the smallest subgroup of interest or the interaction term. For any choice, it is important to be specific about the hypothesis to which each power calculation corresponds.
Whether calculating the smallest detectable effect size for a fixed sample size or the sample size based on an anticipated effect size, it is important to provide a rationale for why the effect size is appropriate. Such a rationale clarifies why an effect at least that large is plausible for the exposure, policy, program, or intervention under study. This justification may be based on the researcher’s theory of change or causal model, and ideally a comparison to effect sizes achieved by previous similar interventions.
If the smallest detectable effect size is large, one concern is that much smaller effects might still be of importance. If smaller but important effects cannot be determined the study will be less informative because a null finding cannot rule out important benefits of the exposure or intervention. E4A primarily seeks to fund studies that are designed in such a manner that either positive or null findings will provide useful information. It is therefore valuable to discuss whether the smallest detectable effect size in your study will be informative, i.e., whether your study is adequately powered to detect the smallest effect size of interest. In a separate Methods Note, we discuss the plausible range of effect sizes for social interventions and what magnitude of effect sizes are worthwhile to study in population health research.
Numerous statistical software packages implement power calculations, including SPSS SamplePower, PASS, GPower, Optimal Design, SAS proc power, the “sampsi” or “power” command in Stata, and the “pwr” package in R. More complex calculations—for example, longitudinal assessments of students clustered within schools—may require adaptations to packaged programs or consultation with a statistician. A helpful introduction to power analysis and further resources can be found here.
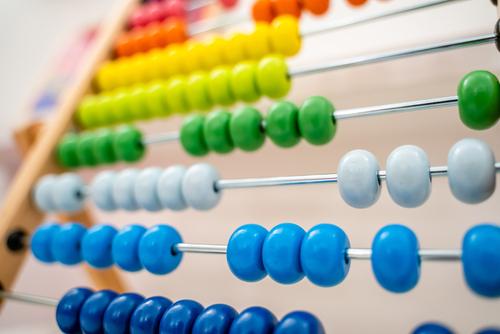

The E4A Methods Lab was developed to address common methods questions or challenges in Culture of Health research. Our goals are to strengthen the research of E4A grantees and the larger community of population health researchers, to help prospective grantees recognize compelling research opportunities, and to stimulate cross-disciplinary conversation and appreciation across the community of population health researchers.
Do you have a suggestion for a future E4A Methods Note? Email us at evidenceforaction@ucsf.edu and let us know.