When the goal of a study is to draw causal inferences about the impact of an intervention on population health outcomes, calculations of statistical power and the related values of sample size and smallest detectable effect size are essential. Often, the most challenging aspect of a power calculation is accurately anticipating what effect sizes are plausible to achieve for the social intervention or exposure under study. Researchers and funders must also consider how large an effect size must be to justify studying a proposed policy or intervention. The purpose of this Methods Note is to discuss considerations for plausible and important effect sizes in population health research.
by Ellicott C. Matthay, PhD
Published January 15, 2020
Plausible Effect Sizes
Effect sizes may be estimated based on pilot studies,1,2 theories of change or causal models, or scientific literature on similar interventions. However, the relevant evidence base for many social interventions remains sparse, leaving population health researchers to guess at likely effects. Cohen’s guidelines3 cite standardized mean differences (“effect sizes”) of 0.20, 0.50, and 0.80 as “small”, “medium”, and “large”, respectively. These benchmarks correspond roughly with the distribution of observed effect sizes in psychology research,4,5 but it is unknown whether they apply to interventions on social conditions. Effect sizes for social interventions are likely to be smaller, because social interventions differ fundamentally from the short-term, proximal outcomes and controlled laboratory settings studied in many psychology experiments.
Historically, even intensive, high-touch, multiyear interventions for high-need populations such as the Nurse-Family Partnership;6,7 highly proximal outcomes such as secondhand smoke exposure for smoke-free air policies;8 and well-established biomedical interventions such as anti-hypertensive medications9 have only reached Cohen’s threshold for a “medium” effect size. In general, effect sizes achieved with social interventions depend on:
- The characteristics of the intervention. Is it an individually-tailored, intensive, or long-term intervention, or a low-intensity population-level intervention such as a state compulsory schooling law? Are there particular nuances or variations in the intervention that may make it more or less effective than those examined in prior research?
- The target population. Is it a high-need population that is likely to benefit substantially, or a general population for which impacts may be more moderate?
- The types of outcomes under study. Are they distal and difficult to shift, like all-cause mortality, or more proximal and mutable, like healthcare utilization?
Population health researchers are likely evaluating programs with “small” or “very small” effects using Cohen’s benchmarks. This implies that large sample sizes are essential and that primary data collection may be unrealistic. Consider the example of compulsory schooling laws.
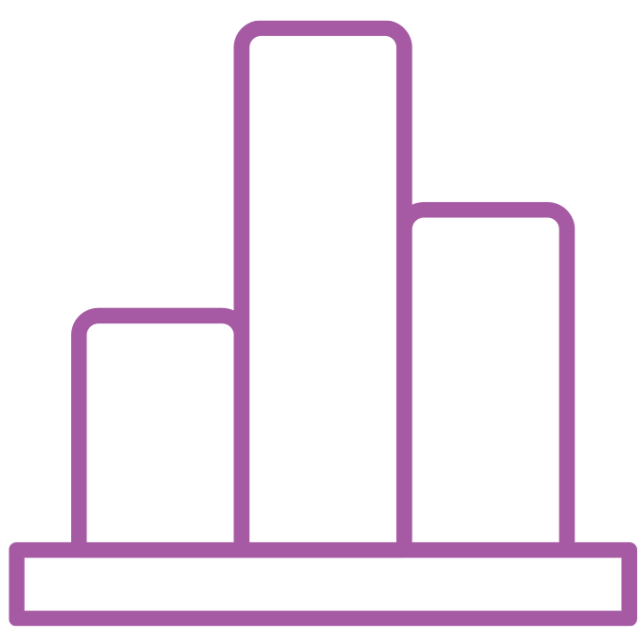
Compulsory Schooling Laws, an Example
Approximating likely effect sizes requires information on both the impact of the social intervention on the presumed mechanism (e.g., how much does an education policy change education?) and the impact of that mechanism on the outcome (e.g., how much do increases in education reduce mortality?). Even when the health effects of the mechanism itself are large, social interventions to modify these exposures are unlikely to shift exposure for everyone and thus correspond to smaller effect sizes.
Educational attainment is believed to have substantial impacts on health and well-being.10,11 Compulsory schooling laws (CSLs) increase educational attainment by requiring a minimum number of years of education among school-age children.12-15 The CSLs can be considered a universal, low-touch, contextual intervention. They involve no individual targeting, tailoring, or person-to-person contact. Most children’s schooling is not determined by the state law because they do not leave school at the earliest legal age. Thus, effects of the law on any population are likely to be relatively small. Still, because of the large populations affected by these laws, CSLs have had important impacts on educational attainment.12-15 Variation in the timing and location of CSL implementation has provided the foundation for numerous studies of the impacts of educational attainment on health.
A recent meta-analysis of the health effects of education, as assessed using CSLs, found that each additional year of schooling was associated with a 5% relative reduction in the adult mortality rate and 20% relative reduction in the lifetime risk of obesity.14 These estimates correspond to approximate effect sizes of 0.03 and 0.16, respectively but because these studies examine education differences induced by CSLs rather than CSLs themselves, they point to the effects of education, not to the effects of CSLs. Given that a one-year increment in a CSL increased average schooling by 0.1 years or less,14 we would expect the effect sizes of CSLs on mortality and obesity to be extremely small — approximately 0.003 and 0.016, respectively—and thus require much larger sample sizes to be detected.
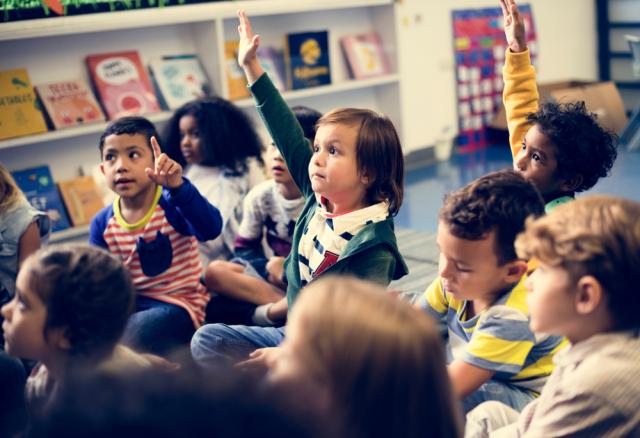
Important Effect Sizes for Population Health
Effect sizes for social interventions are likely to be smaller than Cohen’s benchmarks suggest, yet even very small effect sizes in Cohen’s framework may still be of substantial population health importance. E4A seeks to fund research that is adequately powered to detect any effect size large enough to change population health or health equity. Yet standardized effect sizes alone do not convey this information, because they only contrast outcomes for exposed versus unexposed individuals, without considering what proportion of the population would be exposed to the intervention. The population health impact depends on the proportion exposed as well as the outcome frequency, and heterogeneity in effects of the intervention on different types of people.16 To determine whether a proposed study is worthwhile, researchers and funders must consider the smallest important effect size: i.e., the smallest effect which, if verified, would justify future adoption of the intervention or policy. Every intervention entails both direct costs and opportunity costs. Sample size calculations can therefore also be justified using the smallest important effect size, because demonstrating an intervention had benefits smaller than this threshold would have no actionable implications.
Even a very small effect size might be important for an intervention that could be implemented very broadly with little cost. For example, one E4A-funded study is evaluating the effects of price disclosure on use of health services. This intervention could be broadly implemented for very little cost. Therefore, even a small benefit of the intervention could justify widespread adoption. In contrast, another grant is evaluating a youth development intervention for adolescents, with a fairly intensive program to enhance social and emotional learning. This intervention is likely to be widely adopted only if it has large benefits, so the smallest important effect size is much larger. When evaluating a proposal, E4A considers whether, if the study findings are null, one could conclude that the intervention evaluated is not an important population health lever. Null results are highly informative when they result from studies with adequate power and sample size to detect meaningful effects.
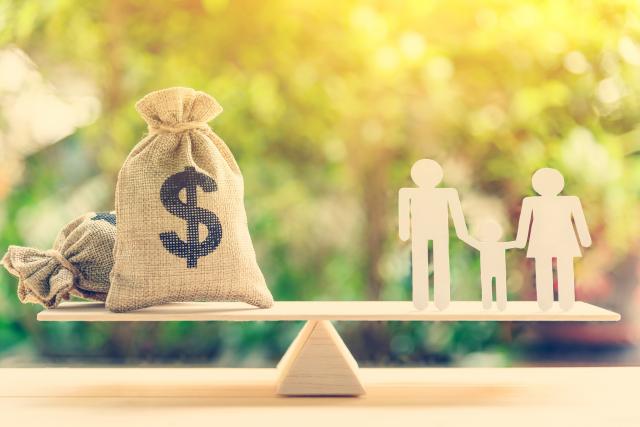
References
- Thabane L, Ma J, Chu R, et al. A tutorial on pilot studies: the what, why and how. BMC Medical Research Methodology. 2010;10(1):1. doi:10.1186/1471-2288-10-1
- Leon AC, Davis LL, Kraemer HC. The role and interpretation of pilot studies in clinical research. Journal of Psychiatric Research. 2011;45(5):626-629. doi:10.1016/j.jpsychires.2010.10.008
- Cohen J. Statistical Power Analysis for the Behavioral Sciences. Second Edition. Hillsdale, New Jersey: Lawrence Erlbaum Associates; 1988.
- Lipsey M, Wilson D. The efficacy of psychological, educational, and behavioral treatment: Confirmation from meta-analysis. American Psychologist. 1993;48(12):1181.
- Sedlmeier P, Gigerenzer G. Do studies of statistical power have an effect on the power of studies? Psychological Bulletin. 1989;105(2):309-316. doi:10.1037/0033-2909.105.2.309
- Olds DL, Robinson J, O’Brien R, et al. Home visiting by paraprofessionals and by nurses: A randomized, controlled trial. Pediatrics. 2002;110(3):486-496. doi:10.1542/peds.110.3.486
- Olds DL, Kitzman H, Cole R, et al. Effects of nurse home-visiting on maternal life course and child development: Age 6 follow-up results of a randomized trial. Pediatrics. 2004;114(6):1550-1559. doi:10.1542/peds.2004-0962
- Community Preventive Services Task Force. Tobacco Use and Secondhand Smoke Exposure: Smoke-Free Policies. The Community Guide; 2014. https://www.thecommunityguide.org/findings/tobacco-use-and-secondhand-smoke-exposure-smoke-free-policies. Accessed February 22, 2019.
- Astell-Burt T, Rowbotham S, Hawe P. Communicating the benefits of population health interventions: The health effects can be on par with those of medication. SSM - Population Health. 2018;6:54-62. doi:10.1016/j.ssmph.2018.06.002
- Campbell F, Conti G, Heckman JJ, et al. Early childhood investments substantially boost adult health. Science. 2014;343(6178):1478-1485. doi:10.1126/science.1248429
- Ross CE, Wu C. The links between education and health. American Sociological Review. 1995;60(5):719-745. doi:10.2307/2096319
- Acemoglu D, Angrist J. How large are the social returns to education? Evidence from compulsory schooling laws. National Bureau of Economic Research; 1999. doi:10.3386/w7444
- Lleras-Muney A. The relationship between education and adult mortality in the United States. The Review of Economic Studies. 2005;72(1):189-221. doi:10.1111/0034-6527.00329
- Hamad R, Elser H, Tran DC, Rehkopf DH, Goodman SN. How and why studies disagree about the effects of education on health: A systematic review and meta-analysis of studies of compulsory schooling laws. Social Science & Medicine. 2018;212:168-178. doi:10.1016/j.socscimed.2018.07.016
- Galama TJ, Lleras-Muney A, van Kippersluis H. The effect of education on health and mortality: A review of experimental and quasi-experimental evidence. National Bureau of Economic Research; 2018. doi:10.3386/w24225
- Min S, Martin LT, Rutter CM, Concannon TW. Are publicly funded health databases geographically detailed and timely enough to support patient-centered outcomes research? Journal of General Internal Medicine. 2019;34(3):467-472. doi:10.1007/s11606-018-4673-6
- Davis JC, Holly BP. Regional analysis using Census Bureau microdata at the Center for Economic Studies. International Regional Science Review. 2006;29(3):278-296. doi:10.1177/0160017606289898
- Erdem E, Korda H, Haffer SC, Sennett C. Medicare claims data as public use files: A new tool for public health surveillance. Journal of Public Health Management and Practice. 2014;20(4):445. doi:10.1097/PHH.0b013e3182a3e958
- Rothman KJ, Greenland S, Lash TL. Modern Epidemiology. Philadelphia, PA: Lippincott Williams & Wilkins; 2008.

The E4A Methods Lab was developed to address common methods questions or challenges in Culture of Health research. Our goals are to strengthen the research of E4A grantees and the larger community of population health researchers, to help prospective grantees recognize compelling research opportunities, and to stimulate cross-disciplinary conversation and appreciation across the community of population health researchers.
We welcome suggestions for new topics for briefs or training areas. Share suggestions with us by emailing evidenceforaction@ucsf.edu.