Introduction
With the push for disaggregation of data across racial/ethnic identities and evaluation of intersectional drivers of health, concerns about the need for multiple comparisons adjustment are common. Multiple comparisons adjustments are typically applied when a large number of statistical tests are made in a sample drawn from a larger population, to improve the likelihood that associations inferred based on the sample data reflect what would be seen in the population. Multiple comparison adjustments are considered best-practice in many genetics studies. In genome-wide association studies (GWAS) for example, statistical adjustments for multiple comparisons protect against overinterpretation of chance associations observed in a sample that would not be observed in the population. A priori hypotheses are not specified in GWAS. Rather, the GWAS may use a sample to evaluate thousands or millions of statistical tests (one for each polymorphism measured) against null hypotheses that each polymorphism has no association with the outcome. This large number of null hypothesis significance tests – especially given that most of the tested associations are expected to be null – necessitates corrections for multiple comparisons in order to avoid type 1 errors (defined as rejecting the null in a sample when no association is present in the population, i.e., overinterpreting chance findings). This reasoning does not necessarily apply to health equity research, however.
Multiple comparisons adjustments entail making a choice between tolerating type 1 errors versus tolerating type 2 errors (defined as failing to reject the null in a sample when an association is present in the population, i.e., missing real associations). Multiple comparisons adjustments intrinsically apply a higher threshold of statistical significance for rejecting a null hypothesis (or equivalently, provide wider confidence intervals). In any finite sample, multiple comparison adjustments thus increase the risk of dismissing real associations as due to chance. In health equity research, such type 2 errors can be harmful, as they equate to hiding inequities. In some cases, multiple comparisons adjustments may still be merited, but they should not be the default for health equity research, even when multiple comparisons are evaluated. Rather, a principled decision on how to prioritize type 1 and type 2 errors should guide this decision. Here, we describe the (mis)use of multiple comparisons adjustments and demonstrate, with a simulation, a hypothetical example of how multiple comparisons adjustments may obscure racial health inequities.
We focus here on null hypothesis significance testing, which has been controversial for many reasons. Most epidemiology now emphasizes interpretation of uncertainty in estimates, e.g., via confidence intervals, instead of p-value thresholds. A multiple comparisons adjustment would widen confidence intervals, however, often to an extent that they are interpreted as offering no useful information. Thus the concerns about multiple comparisons adjustments we describe below in the context of null hypothesis significance testing also apply when research is using other measures of uncertainty, such as confidence intervals.
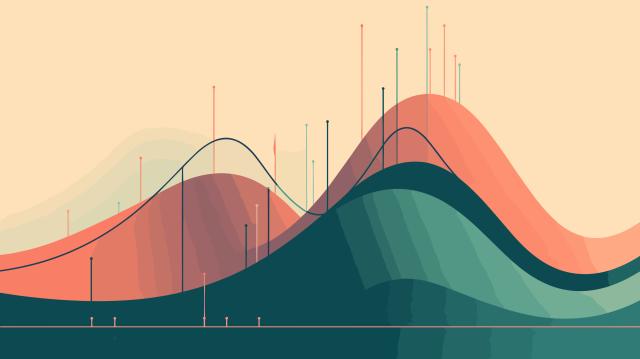
About the Authors
Ye Ji Kim, PhD, is a Postdoctoral Scholar in the E4A Methods Lab.
Peter Buto, MPH, is a Research Fellow at the Boston University School of Public Health.
Amani Nuru-Jeter, PhD, MPH, is the Director of Evidence for Action and Professor of Community Health Sciences and Epidemiology at the University of California, Berkeley, School of Public Health.
Maria Glymour, SD, is an E4A Reviewer and Chair and Professor of Epidemiology at the Boston University School of Public Health.

To Use or Not to Use?
When we conduct more than one statistical test, the chance of at least one association occurring by chance – and being deemed “statistically significant” – increases. The multiple comparison adjustment is important for certain types of studies when a great number of hypotheses, each with very low likelihood of being true, is tested. Multiple comparisons adjustment is intended to keep the likelihood of false positive findings in the study as a whole to a tolerable level.
However, applying multiple comparisons adjustments in the context of health equity research can obscure inequities, making it difficult to identify important differences. A common approach to evaluating differences across multiple racial and ethnic groups begins with a joint test of the null hypothesis that all groups have equivalent health (e.g., Asian, Black, Latine, and White participants all average the same level of health). If the joint test is rejected, we then move on to group-by-group comparisons (e.g., the health of Black participants compared to the health of White participants). It would not then be appropriate to apply a multiple comparisons adjustment to those group-by-group comparisons.
Deciding on applying multiple testing adjustment is more complicated when undertaking single tests of multiple individual null hypotheses without a preceding joint test of the null hypothesis that all groups have equivalent health. When we use a single test to make an inference about a single null hypothesis, the α for that single inference does not become inflated and no multiple testing adjustment is needed. If multiple such inferences are made within the same study and the same dataset, then the probability that at least one association will be discovered by chance – when it would not occur in the population as a whole – increases proportionally. Applying a multiple comparisons correction when trying to interpret specific associations with a larger set of comparisons is often misguided, but a fairly common mistake.
In health equity research, we often have a strong prior that groups have differential health outcomes due to the exposure. With such strong priors, it is often more appropriate to start by characterizing specific group-level differences, rather than with an omnibus test of the joint null. This is in marked contrast to conventional GWAS in which a priori hypotheses are not specified.
The circumstances in which we begin with a joint test or adopt multiple comparisons adjustments should be driven not only by our research question but also by theory and previous literature in which we frame and motivate our research question. For example, differences in well-studied health outcomes between non-Hispanic Black and non-Hispanic White individuals in the US are widespread: a reasonable researcher may begin with a prior expectation that inequities are more likely than not. In such an instance, beginning with a joint test of the null simply makes it less likely to detect the difference of substantive interest. New research questions – for example, about small population subgroups or subgroups defined by intersectional identities – may lack much prior empirical evidence but have substantial theory to help guide hypotheses. If evidence is limited but theory suggests a difference across subgroups is likely, again, those differences should often be assessed directly, without the need to begin with a joint test or apply post-hoc adjustments for multiple comparisons. Rothman suggested that adjustments for multiple comparisons are never needed, as the universal null hypothesis is untenable and other associations in the set of comparisons may have zero bearing on the one in question.
Both joint tests and comparisons adjustments can be used to obscure health inequities. For example, simply disaggregating a single racial/ethnic group into two or more subgroups reduces the statistical power when a joint test or multiple comparisons adjustments are being applied. Thus, in health equity research such approaches merit special critiques. There is no universal rule, but the potential harms to health equity entailed by false positive findings versus false null findings must be considered.
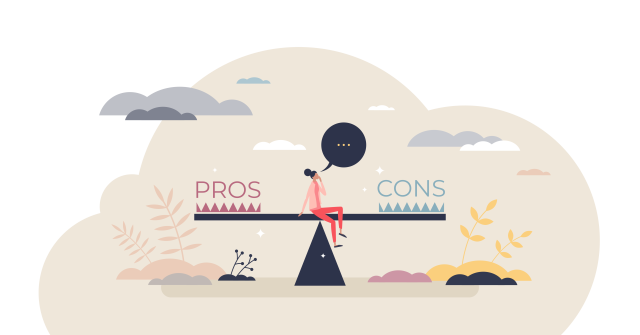

A Hypothetical Example
We illustrate a hypothetical research question to provide a concrete example of how joint tests and multiple comparisons adjustments can obscure important differences. Imagine a situation in which we hypothesize that there are racial and ethnic differences in child food insecurity during the COVID-19 pandemic. We aim to evaluate this hypothesis in a data set of 1,000 individuals (for this simulation, we repeated our analyses over 1,000 iterations of hypothetical samples of 1,000 individuals). We compare the estimate for each of the racial/ethnic groups to a reference category, perhaps the group expected to have the lowest food insecurity. Each comparison to the reference group (e.g., child food insecurity among Black individuals vs. child food insecurity among White individuals) may be well motivated, with strong theoretical or empirical reason to believe differences exist. Further, each comparison may have important substantive implications if group differences are verified, e.g., targeting of child feeding programs. Applying multiple comparisons adjustments could lead to missed associations, suggesting there are no differences in outcomes when, in fact, there are.
We assumed our 1,000 hypothetical individuals identify with one of 4 racial/ethnic groups: non-Hispanic Black, Latine, non-Hispanic White, and other individuals, with sample sizes and mean characteristics in Table 1. We simulated normally distributed variables representing food insecurity, with a mean of -1 and a standard deviation of 1 for Black, Latine, and White individuals. The food insecurity for the “other” group was simulated with a mean of -0.635 and standard deviation of 1 to allow for detection of group difference in the outcome. The “other” race/ethnicity group is first tested in Model 1 as a single group (n=100), whereas in Model 2, we further break down the group to Asians and Pacific Islanders (n=70; API) and Indigenous Peoples (n=30), creating even smaller subgroups. Note that in the simulation, both API and Indigenous Peoples average higher food insecurity than other groups.
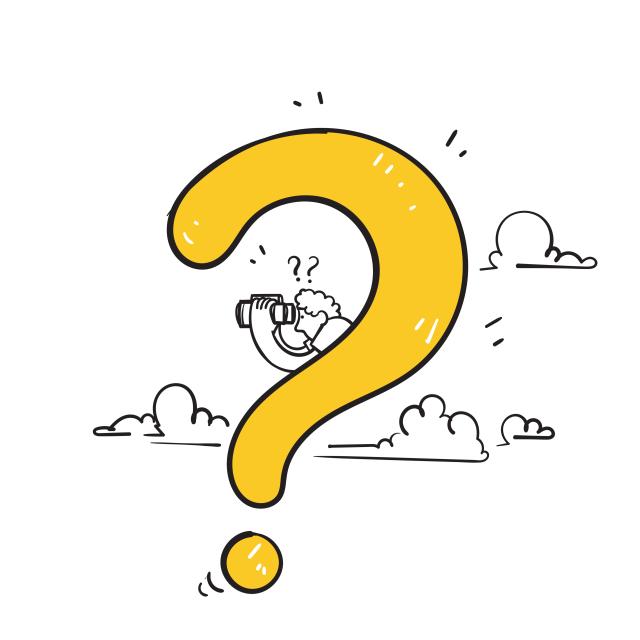
Table 1. Sample sizes and summary outcome statistics of simulated population of N = 1000 by race/ethnicity: IQR, median, mean, standard deviation, and standard error of the mean across 1,000 iterations
Race/Ethnicity | N | IQR | Median | Mean | SD | SEM |
---|---|---|---|---|---|---|
White, NH | 430 | 1.35 | -1.00 | -1.00 | 1.00 | 0.03 |
Black, NH | 320 | 1.34 | -1.00 | -1.00 | 1.00 | 0.03 |
Latine | 150 | 1.34 | -1.00 | -1.00 | 1.00 | 0.03 |
Other | 100 | 1.33 | -0.63 | -0.64 | 1.00 | 0.03 |
API, NH | 70 | 1.33 | -0.64 | -0.64 | 1.00 | 0.03 |
Indigenous Peoples, NH | 30 | 1.29 | -0.63 | -0.64 | 0.99 | 0.03 |
Note: Means for White, Black and Latine were set as -1. For the other category, the mean was set to -0.635. SDs were set as 1 for all groups under the normal distribution for the simulation. Summary statistics values shown are mean values across 1,000 iterations of n = 1000.
Abbreviations: NH = non-Hispanic; IQR = interquartile range; SD = standard deviation; SEM = standard error of the mean; API = Asian and Pacific Islanders
Table 2 provides the joint statistic testing the null hypothesis that food insecurity is similar across all race and ethnic groups, the model estimates from a linear regression, and accompanying p-values. The p-values listed include the nominal, then p-values adjusted for multiple comparisons using Bonferroni and Benjamini-Hochberg methods. The tests of the joint null hypotheses (that all coefficients are the same across racial and ethnic groups) meets the conventional statistical significance threshold in Model 1 when only 4 groups are considered (p=0.04), but not in model 2, where the same data is disaggregated to allow specific evaluation of API and Indigenous individuals (p=0.05). Note that the joint test does not allow us to specify which race and ethnicity had a significant difference in food insecurity; it simply tells us that the outcomes were different for one or more groups. Based on the joint test, while we would conclude that there was a differential relationship for one or more groups in the first model, we would conclude the opposite based on the joint test in Model 2. If we further disaggregated the other groups, for example considering separate Latinx/e subgroups, the statistical power of the joint test would further deteriorate.
Table 2. Model Summary Statistics and Comparison of Nominal to Multiple Comparison Adjusted p-values, iterated 1,000 times
Joint Test F-statistic | Joint Test p | Race/Ethnicity | Estimate | SE | Nominal p | Adjusted p | |
Bonferroni | BH | ||||||
Model 1: 4 race categories (3 indicator variables for race/ethnicity compared to White, NH) as independent variables in linear regression of outcome y | |||||||
4.95a | 0.04 | Black, NH | 0.00 | 0.07 | 0.50 | 0.84 | 0.68 |
Latine | 0.00 | 0.09 | 0.50 | 0.83 | 0.67 | ||
Other, NH | 0.36 | 0.11 | 0.02 | 0.05 | 0.05 | ||
Model 2: 5 race categories (4 indicator variables for race/ethnicity compared to White, NH) as independent variables in linear regression of outcome y | |||||||
3.96b | 0.05 | Black, NH | 0.00 | 0.07 | 0.50 | 0.88 | 0.71 |
Latine | 0.00 | 0.09 | 0.50 | 0.88 | 0.71 | ||
API, NH | 0.36 | 0.13 | 0.04 | 0.13 | 0.11 | ||
Indigenous Peoples, NH | 0.36 | 0.19 | 0.17 | 0.41 | 0.33 |
Note: All model values shown are mean values across 1,000 iterations of n = 1000. a Degrees of freedom = F(3, 996). b Degrees of freedom = F(4, 995).
Abbreviations: NH = non-Hispanic; SE = standard error; BH = Benjamini-Hochberg; API = Asian and Pacific Islanders
If we were to make statistical inferences about each of the hypotheses separately (e.g., compare food insecurity among non-Hispanic Black vs. non-Hispanic White), the inferences will be based on tests of individual null hypotheses (e.g., Black and White children have equal average levels of food insecurity) and do not require a multiple testing adjustment. Such inferences are common, as we are often interested in the impact of policies and interventions within racial/ethnic groups, neighborhoods, or counties of interest.
In model 1, the nominal p-value for the “other” race/ethnicity group (p=0.02) indicates that the average in that group differs from the average for the non-Hispanic White group. In model 2, the nominal p-values for API individuals (p=0.04) also indicates that API children average higher food insecurity than children in the non-Hispanic White group. However, the nominal p-value for Indigenous people is not significant, and a conservative reading of this result would force the conclusion of no evidence that Indigenous children were averaging worse food insecurity than non-Hispanic White children.
Further, adjustment of Model 2 results for multiple comparisons results in non-significant p-values for both API and Indigenous groups, suggesting the food insecurity differences for both groups compared to White individuals are likely attributable to chance. Both the joint test (which moves from 0.04 to 0.05) and the multiple comparisons adjustments of p-values serve to obscure racial and ethnic differences when the data are disaggregated. After adjusting for multiple comparisons, we would incorrectly conclude that food insecurity for API individuals was no different from that of non-Hispanic White individuals. In fact, any inequity could be “hidden” with enough data disaggregation. Although not addressed here to maintain focus on the current topic, similar concerns are relevant for effect modifiers assessed via interactions in statistical models (e.g., policy effects).
In research, prioritization of our research questions and tolerance for over-looking important differences versus over-interpreting chance associations must drive the methods we choose. If we have a strong prior belief that one or more racial and ethnic groups are likely to experience inequity in child food insecurity, it would be misleading to limit the evaluation of these differences based on a joint test prior to subgroup-specific comparisons or to make it harder to detect differences by applying multiple comparisons adjustments.
R code to simulate the hypothetical example is available on GitHub. The code simulates 1,000 individuals which is iterated 1,000 times, then calculates mean estimates across the iterations. We encourage you to download the code and change model parameters and sample characteristics to understand how they impact the study conclusion.
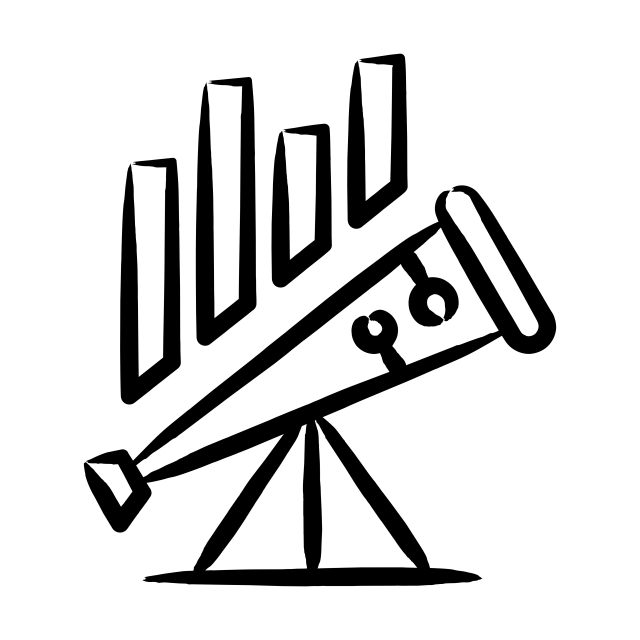

Concluding Thoughts
Health equity research already struggles to overcome statistical power limitations due to inherently small sample sizes, especially when considering intersectional identities. By gratuitously adjusting for multiple testing, we are further restricting our ability to understand differential impacts on systemically excluded groups. Adding more groups to compare, as in intersectional identity groups – for example, gender, sexual identity, ethnicity, socioeconomic status, and religion – will typically reduce statistical power, increasing the chances that we overlook important differences.
The major concern motivating multiple comparisons adjustments is to avoid overinterpreting chance findings. Our research question should serve as the fundamental base guiding the selection of our methods, necessitating a clear and accurate identification and reporting of the need for multiple comparison adjustments, if and when used. Indeed, “science comprises a multitude of comparisons, and this simple fact in itself is no cause for alarm.” But determining whether, when, and how to correct for these multiple comparisons – guided by a nuanced understanding of the strength of prior empirical or theoretical evidence and the relative harms of false positive versus false null results – is essential.
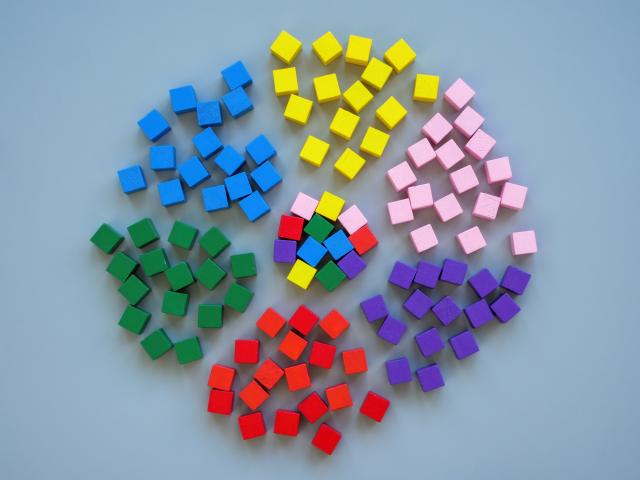

- Rubin, M. (2024). Redundant multiple testing corrections: The fallacy of using family-based error rates to make inferences about individual hypotheses. Methods in Psychology, 10, 100140. https://arxiv.org/abs/2401.11507
- García-Pérez, M. A. (2023). Use and misuse of corrections for multiple testing. Methods in Psychology, 8, 100120. https://doi.org/10.1016/j.metip.2023.100120
- Kauh, T. J. (2021). Racial equity will not be achieved without investing in data disaggregation. Health Affairs Forefront. 10.1377/forefront.20211123.426054
- Midway, S., Robertson, M., Flinn, S., & Kaller, M. (2020). Comparing multiple comparisons: Practical guidance for choosing the best multiple comparisons test. PeerJ, 8, e10387. https://doi.org/10.7717/peerj.10387
- Rothman, K. J. (1990). No adjustments are needed for multiple comparisons. Epidemiology, 1(1), 43-46.