Instrument-based study designs are powerful tools to evaluate the causal effects of a treatment or exposure on a health outcome1 (see also “Evidence for Action Methods Note: Confounder-Control Versus Instrumental Variables”). Many instrument-based study designs can be described as “quasi-experimental”, although this term has been used inconsistently in prior research. These designs share characteristics with experimental randomized studies: in both experimental and instrument-based designs, researchers exploit some “exogenous”, plausibly random source of variation in the treatment. In experimental studies, this exogenous factor is often random assignment to treatment by the investigator, and in instrument-based studies, this exogenous factor is often a “quasi-random” change in a program or policy or an accident of time and space. To make causal inferences, both designs require a key assumption to be true: the exogenous factor must influence treatment but have no other plausible reason to be associated with the outcome. When this assumption is met, the statistical associations between the exogenous factor, the treatment, and the outcome can then be used to estimate the causal effect of the treatment on the outcome.
Several closely related instrument-based methods have different names, such as instrumental variables, differences-in-differences, or fuzzy regression discontinuity analyses. Each can be analyzed using the statistical methods for instrumental variables, assuming that the exposure of interest has been measured. Understanding how these methods differ and why one might be preferred over another can make it easier to decide which, if any, are applicable and valid in specific contexts.
by Ellicott C. Matthay, PhD
Published December 19, 2019
1. Angrist JD, Pischke J-S. Mostly Harmless Econometrics: An Empiricist’s Companion. Princeton University Press 2008.
Instrumental Variables Designs
Instrumental variables (IV) designs are common, and the ideas for IV extend easily to other approaches. Consider the example of offenders arbitrarily assigned to judges. Judges may have different propensities for leniency. Judge assignment is plausibly random and is an example of an “instrument” that affects sentencing to probation versus jail time (a potential treatment of interest) and in turn health (the outcome). IV analyses assume that some people are the type who will be affected by the instrument, while others are not. For example, some may receive jail time regardless of the judge assigned, while for others, judge assignment matters.
Figure 1
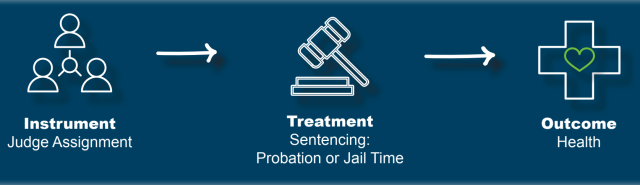
One cannot tell which people are which type, but the researcher can assume that all types are equally likely to be assigned to any judge. The effect of probation versus jail time on health among affected individuals can then be estimated as the ratio of the effect of judge assignment on health to the effect of the judge assignment on the sentence. Intuitively, this works because, for any sentence, average differences in health between people whose sentence was affected versus unaffected by judge assignment must be due to the “affected” type and thus due to the sentence.
In some cases, the instrument may be imperfect. For example, high-income individuals may manipulate their assignment to more lenient judges and also have better health outcomes. This pattern would threaten the validity of the IV estimates, because judge assignment would therefore be related to health but not through the sentence. Statistical control for household income can then effectively recover the instrument-based study. At least in sensitivity analyses, most IV analyses control for some variables that threaten the validity of the instrument.
Fuzzy Regression Discontinuity Design
In a fuzzy regression discontinuity (RD) design, people are assigned to treatment if they are above a specific threshold which would otherwise be unrelated to health. For example, means-tested policies set an arbitrary income threshold to provide some resource; if the resource has health consequences, the health of people right below the income threshold should differ from the health of people right above the threshold. Policies with age-eligibility thresholds, such as Medicare, school enrollment, or work permits, create similar arbitrary thresholds. People on either side of the age-limit should otherwise be very similar, but the age-eligible group is much more likely to be treated. We can use this essentially arbitrary discontinuity in the likelihood of receiving treatment to estimate the effect of receiving the treatment on health. Of course, some people may find a workaround to the eligibility requirements and somehow attain treatment even if they are technically ineligible or avoid treatment even if they are eligible. This “fuzziness” at the threshold can be accounted for using the same methods as for IV. Residual differences in the characteristics of treated and untreated groups that matter for the outcome (e.g., a few months of age for children or a difference of only a few hundred dollars in annual income for families) can be addressed through statistical adjustment.
The date when a policy or program regulating the treatment was introduced is an appealing source of a regression discontinuity. For example, if a policy to reduce air pollution went into effect in one community on January 1980, the effect of air pollution on health of the community could be estimated by comparing the health of the same community members before and after January 1980. This approach is even stronger if we have multiple measures of health leading up to the policy change and multiple measures after the policy change, a design called an interrupted time series (ITS). The key assumption for RD or ITS based on the timing of a new policy is that nothing else happened on or around that date, such as another policy change or a major historical event, which might affect health in the community. The differences-in-differences (DiD) design accounts for the possibility that there was another influential event by comparing the difference in health before and after the policy change (difference #1) to the difference in health across the same time frame for a comparable community that did not introduce the policy (difference #2). The second community serves as a comparison or negative control. If air pollution levels before and after the policy introduction data have been measured, data from either a regression discontinuity or differences-in-differences design can be analyzed using the same statistical methods as an IV, where the instrument is time (in the case of RD) or the interaction of time and place (in the case of DiD).
Instrument-based designs based on the introduction of new programs or policies can be used to answer two different types of research questions:
- What is the effect of the program or policy on the health of the population as a whole?
- What is the effect of the resource or treatment regulated by the program policy on people who receive the resource or treatment as a result?
Although E4A is always interested in the effects of programs and policies, both types of questions are usually of interest. The distinction depends on how closely aligned the program or policy is with the delivery of resources. In cases of close alignment, as in a program to provide home weatherization to low-income households, instrument-based estimates of the health effect of the program and the health effect of the treatment delivered will be very similar. In cases of poor alignment, as in a study of the health effects of additional income induced by living wage policies, the effect of the policy itself on the health of the population may be quite different from the health effect among those who actually receive additional income due to the policy. We often want to know the effects of the resources, treatments, or exposures regulated by programs or policies, because many alternative policies may affect the same resources. Thus, knowing the effects of the resource allows us to predict the potential health benefits of these alternative policies. The overall effect of any policy is partly dependent on how many people are influenced by the policy, which may depend on the context—for example, how many people became eligible because of the policy change or how the policy was enforced. These factors may change in different settings. Therefore, knowing the effects of the resultant resources, treatments, or exposures is useful, in addition to knowing the effects of specific programs or policies.
When can these designs deliver convincing evidence on how to improve population health? For each setting, this depends on the alternative explanations that need to be ruled out. For example, threats such as concurrent events may be especially worrisome for RD studies based on the timing of a new policy’s introduction. All of these designs (IV, RD, ITS, and DiD) share the assumption that an apparently random or arbitrary factor (e.g., the instrumental variable or the date of a policy change) influences the treatment but has no other reason to be associated with the outcome except via its effect on the treatment. Beyond this, alternative explanations have to be identified and ruled out on a case-by-case basis using detailed understanding of how the system works. In evaluating E4A grant applications, we consider the plausibility of assumptions and potential threats to validity.

The E4A Methods Lab was developed to address common methods questions or challenges in Culture of Health research. Our goals are to strengthen the research of E4A grantees and the larger community of population health researchers, to help prospective grantees recognize compelling research opportunities, and to stimulate cross-disciplinary conversation and appreciation across the community of population health researchers. We welcome suggestions for new topics for briefs or training areas.
Have an idea for what should be covered in a future E4A Methods Note? Send us an email at evidenceforaction@ucsf.edu and let us know.